Evaluate pretrained embeddings#
%config InlineBackend.figure_format='retina'
import warnings
import logging
from ekorpkit import eKonf
logging.basicConfig(level=logging.INFO)
warnings.filterwarnings('ignore')
print(eKonf.__version__)
0.1.32+1.gcf6615f.dirty
data_dir = "../data/embeddings"
save_dataframe = eKonf.partial(
config_group="_func_/save_dataframe", output_dir=data_dir
)
load_dataframe = eKonf.partial(config_group="_func_/load_dataframe", data_dir=data_dir)
Load GloVE Vectors#
Wikipedia#
cfg = eKonf.compose(config_group="model/embedding")
cfg.name = "glove_wiki"
cfg.corpus = "Wikipedia"
cfg.cache.uri = "https://nlp.stanford.edu/data/glove.6B.zip"
cfg.model_dir = cfg.cache.path
cfg.model_file = "glove.6B.300d.txt"
cfg.model_type = "glove"
wv_wiki = eKonf.instantiate(cfg)
wv_wiki.load()
INFO:cached_path:cache of https://nlp.stanford.edu/data/glove.6B.zip is up-to-date
INFO:ekorpkit.io.cached_path:cached path: /workspace/.cache/cached_path/d97dcf99e9ed61e3b07a5a87dd5dea7b6a4815ba6eb1ac87f529bfe6e2ba4ccf.f9662ccb99e715467e6da0c85a047cfc51888321958f9440eb5a15f7189c140f-extracted
INFO:cached_path:cache of https://nlp.stanford.edu/data/glove.6B.zip is up-to-date
INFO:ekorpkit.io.cached_path:cached path: /workspace/.cache/cached_path/d97dcf99e9ed61e3b07a5a87dd5dea7b6a4815ba6eb1ac87f529bfe6e2ba4ccf.f9662ccb99e715467e6da0c85a047cfc51888321958f9440eb5a15f7189c140f-extracted
INFO:cached_path:cache of https://raw.githubusercontent.com/RaRe-Technologies/gensim/develop/gensim/test/test_data/questions-words.txt is up-to-date
INFO:ekorpkit.io.cached_path:cached path: /root/.ekorpkit/.cache/cached_path/7568e1428a2ba53a33f67dea7e276f212c065b07d102aafc177a507d244161f6.f60f7f82aaacf3a534d93013617bcfead46a795268096ff1990608b16115b566
INFO:gensim.models.keyedvectors:loading projection weights from /workspace/.cache/cached_path/d97dcf99e9ed61e3b07a5a87dd5dea7b6a4815ba6eb1ac87f529bfe6e2ba4ccf.f9662ccb99e715467e6da0c85a047cfc51888321958f9440eb5a15f7189c140f-extracted/glove.6B.300d.txt
INFO:gensim.utils:KeyedVectors lifecycle event {'msg': 'loaded (400000, 300) matrix of type float32 from /workspace/.cache/cached_path/d97dcf99e9ed61e3b07a5a87dd5dea7b6a4815ba6eb1ac87f529bfe6e2ba4ccf.f9662ccb99e715467e6da0c85a047cfc51888321958f9440eb5a15f7189c140f-extracted/glove.6B.300d.txt', 'binary': False, 'encoding': 'utf8', 'datetime': '2022-06-08T10:14:23.606212', 'gensim': '4.2.0', 'python': '3.8.12 (default, Jan 14 2022, 01:33:56) \n[GCC 7.5.0]', 'platform': 'Linux-5.4.0-58-generic-x86_64-with-glibc2.27', 'event': 'load_word2vec_format'}
wiki_results = wv_wiki.evaluate_word_analogies(analogies="google")
wiki_results.keys()
INFO:gensim.models.keyedvectors:Evaluating word analogies for top 300000 words in the model on /root/.ekorpkit/.cache/cached_path/7568e1428a2ba53a33f67dea7e276f212c065b07d102aafc177a507d244161f6.f60f7f82aaacf3a534d93013617bcfead46a795268096ff1990608b16115b566
INFO:gensim.models.keyedvectors:capital-common-countries: 94.9% (480/506)
INFO:gensim.models.keyedvectors:capital-world: 96.0% (4342/4524)
INFO:gensim.models.keyedvectors:currency: 17.1% (138/808)
INFO:gensim.models.keyedvectors:city-in-state: 59.3% (1463/2467)
INFO:gensim.models.keyedvectors:family: 88.1% (446/506)
INFO:gensim.models.keyedvectors:gram1-adjective-to-adverb: 22.6% (224/992)
INFO:gensim.models.keyedvectors:gram2-opposite: 27.3% (222/812)
INFO:gensim.models.keyedvectors:gram3-comparative: 88.1% (1174/1332)
INFO:gensim.models.keyedvectors:gram4-superlative: 72.2% (810/1122)
INFO:gensim.models.keyedvectors:gram5-present-participle: 70.0% (739/1056)
INFO:gensim.models.keyedvectors:gram6-nationality-adjective: 92.6% (1480/1599)
INFO:gensim.models.keyedvectors:gram7-past-tense: 61.2% (954/1560)
INFO:gensim.models.keyedvectors:gram8-plural: 78.1% (1040/1332)
INFO:gensim.models.keyedvectors:gram9-plural-verbs: 58.5% (509/870)
INFO:gensim.models.keyedvectors:Quadruplets with out-of-vocabulary words: 0.3%
INFO:gensim.models.keyedvectors:NB: analogies containing OOV words were skipped from evaluation! To change this behavior, use "dummy4unknown=True"
INFO:gensim.models.keyedvectors:Total accuracy: 72.0% (14021/19486)
INFO:ekorpkit.models.embeddings.wordvec:Evaluation score: 0.7195422354510931
dict_keys(['score', 'summary', 'correct', 'incorrect'])
Twitter Data#
cfg = eKonf.compose(config_group="model/embedding")
cfg.name = "glove_twitter"
cfg.corpus = "Twitter"
cfg.cache.uri = "https://nlp.stanford.edu/data/glove.twitter.27B.zip"
cfg.model_dir = cfg.cache.path
cfg.model_file = "glove.twitter.27B.200d.txt"
cfg.model_type = "glove"
wv_twt = eKonf.instantiate(cfg)
wv_twt.load()
INFO:cached_path:cache of https://nlp.stanford.edu/data/glove.twitter.27B.zip is up-to-date
INFO:ekorpkit.io.cached_path:cached path: /workspace/.cache/cached_path/d08f2221566196fe3fd6f557a2b67e9ffd5cf4b7c500918d98581a52f349a804.7417464dae8ba25e69e639021d320765d2d18bb24f73391ef423756069dc8078-extracted
INFO:cached_path:cache of https://nlp.stanford.edu/data/glove.twitter.27B.zip is up-to-date
INFO:ekorpkit.io.cached_path:cached path: /workspace/.cache/cached_path/d08f2221566196fe3fd6f557a2b67e9ffd5cf4b7c500918d98581a52f349a804.7417464dae8ba25e69e639021d320765d2d18bb24f73391ef423756069dc8078-extracted
INFO:cached_path:cache of https://raw.githubusercontent.com/RaRe-Technologies/gensim/develop/gensim/test/test_data/questions-words.txt is up-to-date
INFO:ekorpkit.io.cached_path:cached path: /root/.ekorpkit/.cache/cached_path/7568e1428a2ba53a33f67dea7e276f212c065b07d102aafc177a507d244161f6.f60f7f82aaacf3a534d93013617bcfead46a795268096ff1990608b16115b566
INFO:gensim.models.keyedvectors:loading projection weights from /workspace/.cache/cached_path/d08f2221566196fe3fd6f557a2b67e9ffd5cf4b7c500918d98581a52f349a804.7417464dae8ba25e69e639021d320765d2d18bb24f73391ef423756069dc8078-extracted/glove.twitter.27B.200d.txt
INFO:gensim.utils:KeyedVectors lifecycle event {'msg': 'loaded (1193514, 200) matrix of type float32 from /workspace/.cache/cached_path/d08f2221566196fe3fd6f557a2b67e9ffd5cf4b7c500918d98581a52f349a804.7417464dae8ba25e69e639021d320765d2d18bb24f73391ef423756069dc8078-extracted/glove.twitter.27B.200d.txt', 'binary': False, 'encoding': 'utf8', 'datetime': '2022-06-08T09:15:08.452346', 'gensim': '4.2.0', 'python': '3.8.12 (default, Jan 14 2022, 01:33:56) \n[GCC 7.5.0]', 'platform': 'Linux-5.4.0-58-generic-x86_64-with-glibc2.27', 'event': 'load_word2vec_format'}
twt_results = wv_twt.evaluate_word_analogies(analogies="google")
twt_results.keys()
INFO:gensim.models.keyedvectors:Evaluating word analogies for top 300000 words in the model on /root/.ekorpkit/.cache/cached_path/7568e1428a2ba53a33f67dea7e276f212c065b07d102aafc177a507d244161f6.f60f7f82aaacf3a534d93013617bcfead46a795268096ff1990608b16115b566
INFO:gensim.models.keyedvectors:capital-common-countries: 70.6% (357/506)
INFO:gensim.models.keyedvectors:capital-world: 74.6% (1639/2198)
INFO:gensim.models.keyedvectors:currency: 3.3% (15/458)
INFO:gensim.models.keyedvectors:city-in-state: 35.6% (879/2467)
INFO:gensim.models.keyedvectors:family: 79.5% (302/380)
INFO:gensim.models.keyedvectors:gram1-adjective-to-adverb: 12.7% (118/930)
INFO:gensim.models.keyedvectors:gram2-opposite: 30.8% (185/600)
INFO:gensim.models.keyedvectors:gram3-comparative: 74.2% (989/1332)
INFO:gensim.models.keyedvectors:gram4-superlative: 64.0% (718/1122)
INFO:gensim.models.keyedvectors:gram5-present-participle: 66.4% (701/1056)
INFO:gensim.models.keyedvectors:gram6-nationality-adjective: 72.7% (894/1229)
INFO:gensim.models.keyedvectors:gram7-past-tense: 53.1% (787/1482)
INFO:gensim.models.keyedvectors:gram8-plural: 76.0% (1012/1332)
INFO:gensim.models.keyedvectors:gram9-plural-verbs: 52.0% (452/870)
INFO:gensim.models.keyedvectors:Quadruplets with out-of-vocabulary words: 18.3%
INFO:gensim.models.keyedvectors:NB: analogies containing OOV words were skipped from evaluation! To change this behavior, use "dummy4unknown=True"
INFO:gensim.models.keyedvectors:Total accuracy: 56.7% (9048/15962)
INFO:ekorpkit.models.embeddings.wordvec:Evaluation score: 0.5668462598671845
dict_keys(['score', 'summary', 'correct', 'incorrect'])
Common Crawl#
cfg = eKonf.compose(config_group="model/embedding")
cfg.name = "glove_commoncrawl"
cfg.corpus = "Common Crawl"
cfg.cache.uri = "https://nlp.stanford.edu/data/glove.42B.300d.zip"
cfg.model_dir = cfg.cache.path
cfg.model_file = "glove.42B.300d.txt"
cfg.model_type = "glove"
wv_cc = eKonf.instantiate(cfg)
wv_cc.load()
INFO:cached_path:cache of https://nlp.stanford.edu/data/glove.42B.300d.zip is up-to-date
INFO:ekorpkit.io.cached_path:cached path: /workspace/.cache/cached_path/1b77e307d0976680276bd5eddffac55a83164787611dccb81aaaab9c4c79073b.d4304a004cdd3b0267ad688b5fc4d6c9d43c622a571ee2ba8b39604fc257faed-extracted
INFO:cached_path:cache of https://nlp.stanford.edu/data/glove.42B.300d.zip is up-to-date
INFO:ekorpkit.io.cached_path:cached path: /workspace/.cache/cached_path/1b77e307d0976680276bd5eddffac55a83164787611dccb81aaaab9c4c79073b.d4304a004cdd3b0267ad688b5fc4d6c9d43c622a571ee2ba8b39604fc257faed-extracted
INFO:cached_path:cache of https://raw.githubusercontent.com/RaRe-Technologies/gensim/develop/gensim/test/test_data/questions-words.txt is up-to-date
INFO:ekorpkit.io.cached_path:cached path: /root/.ekorpkit/.cache/cached_path/7568e1428a2ba53a33f67dea7e276f212c065b07d102aafc177a507d244161f6.f60f7f82aaacf3a534d93013617bcfead46a795268096ff1990608b16115b566
INFO:gensim.models.keyedvectors:loading projection weights from /workspace/.cache/cached_path/1b77e307d0976680276bd5eddffac55a83164787611dccb81aaaab9c4c79073b.d4304a004cdd3b0267ad688b5fc4d6c9d43c622a571ee2ba8b39604fc257faed-extracted/glove.42B.300d.txt
INFO:gensim.utils:KeyedVectors lifecycle event {'msg': 'loaded (1917494, 300) matrix of type float32 from /workspace/.cache/cached_path/1b77e307d0976680276bd5eddffac55a83164787611dccb81aaaab9c4c79073b.d4304a004cdd3b0267ad688b5fc4d6c9d43c622a571ee2ba8b39604fc257faed-extracted/glove.42B.300d.txt', 'binary': False, 'encoding': 'utf8', 'datetime': '2022-06-08T09:22:21.176032', 'gensim': '4.2.0', 'python': '3.8.12 (default, Jan 14 2022, 01:33:56) \n[GCC 7.5.0]', 'platform': 'Linux-5.4.0-58-generic-x86_64-with-glibc2.27', 'event': 'load_word2vec_format'}
cc_results = wv_cc.evaluate_word_analogies(analogies="google")
cc_results.keys()
INFO:gensim.models.keyedvectors:Evaluating word analogies for top 300000 words in the model on /root/.ekorpkit/.cache/cached_path/7568e1428a2ba53a33f67dea7e276f212c065b07d102aafc177a507d244161f6.f60f7f82aaacf3a534d93013617bcfead46a795268096ff1990608b16115b566
INFO:gensim.models.keyedvectors:capital-common-countries: 95.1% (481/506)
INFO:gensim.models.keyedvectors:capital-world: 94.0% (4178/4446)
INFO:gensim.models.keyedvectors:currency: 17.6% (142/808)
INFO:gensim.models.keyedvectors:city-in-state: 78.1% (1926/2467)
INFO:gensim.models.keyedvectors:family: 90.9% (460/506)
INFO:gensim.models.keyedvectors:gram1-adjective-to-adverb: 30.2% (300/992)
INFO:gensim.models.keyedvectors:gram2-opposite: 35.6% (289/812)
INFO:gensim.models.keyedvectors:gram3-comparative: 85.6% (1140/1332)
INFO:gensim.models.keyedvectors:gram4-superlative: 84.0% (942/1122)
INFO:gensim.models.keyedvectors:gram5-present-participle: 80.9% (854/1056)
INFO:gensim.models.keyedvectors:gram6-nationality-adjective: 88.3% (1412/1599)
INFO:gensim.models.keyedvectors:gram7-past-tense: 49.3% (769/1560)
INFO:gensim.models.keyedvectors:gram8-plural: 84.9% (1131/1332)
INFO:gensim.models.keyedvectors:gram9-plural-verbs: 64.1% (558/870)
INFO:gensim.models.keyedvectors:Quadruplets with out-of-vocabulary words: 0.7%
INFO:gensim.models.keyedvectors:NB: analogies containing OOV words were skipped from evaluation! To change this behavior, use "dummy4unknown=True"
INFO:gensim.models.keyedvectors:Total accuracy: 75.1% (14582/19408)
INFO:ekorpkit.models.embeddings.wordvec:Evaluation score: 0.7513396537510305
dict_keys(['score', 'summary', 'correct', 'incorrect'])
Evaluate embeddings#
import pandas as pd
eval_summary = pd.concat(
[wiki_results["summary"], twt_results["summary"], cc_results["summary"]]
)
save_dataframe(eval_summary, output_file="eval_summary.parquet")
INFO:ekorpkit.io.file:Saving dataframe as ../data/embeddings/eval_summary.parquet
eval_summary = load_dataframe(data_file="eval_summary.parquet")
eval_summary.head()
INFO:ekorpkit.io.file:Loading data from ../data/embeddings/eval_summary.parquet
Category | Correct | Incorrect | Samples | Average | Corpus | |
---|---|---|---|---|---|---|
0 | Capitals | 480 | 26 | 506 | 0.948617 | Wikipedia |
1 | Capitals RoW | 4342 | 182 | 4524 | 0.959770 | Wikipedia |
2 | Currency | 138 | 670 | 808 | 0.170792 | Wikipedia |
3 | City-State | 1463 | 1004 | 2467 | 0.593028 | Wikipedia |
4 | Famliy | 446 | 60 | 506 | 0.881423 | Wikipedia |
cfg = eKonf.compose(config_group="visualize/plot=barplot")
cfg.plots[0].x = "Category"
cfg.plots[0].y = "Average"
cfg.plots[0].hue = "Corpus"
cfg.ax.ytickmajorformatterfunc = "lambda y, _: '{:.0%}'.format(y)"
cfg.ax.ylabel = "Accuracy"
cfg.figure.figsize = (16, 5)
cfg.figure.fontsize = 10
cfg.ax.title = f"Word Vector Accuracy by Glove Source: Twitter: {twt_results['score']:.2%}, Wiki: {wiki_results['score']:.2%}, Crawl: {cc_results['score']:.2%}"
eKonf.instantiate(cfg, data=eval_summary)
INFO:ekorpkit.visualize.plot:Plotting barplot with {'x': 'Category', 'y': 'Average', 'hue': 'Corpus'}
INFO:ekorpkit.visualize.plot:Saved figure to ./figs/plot_BarPlot.png
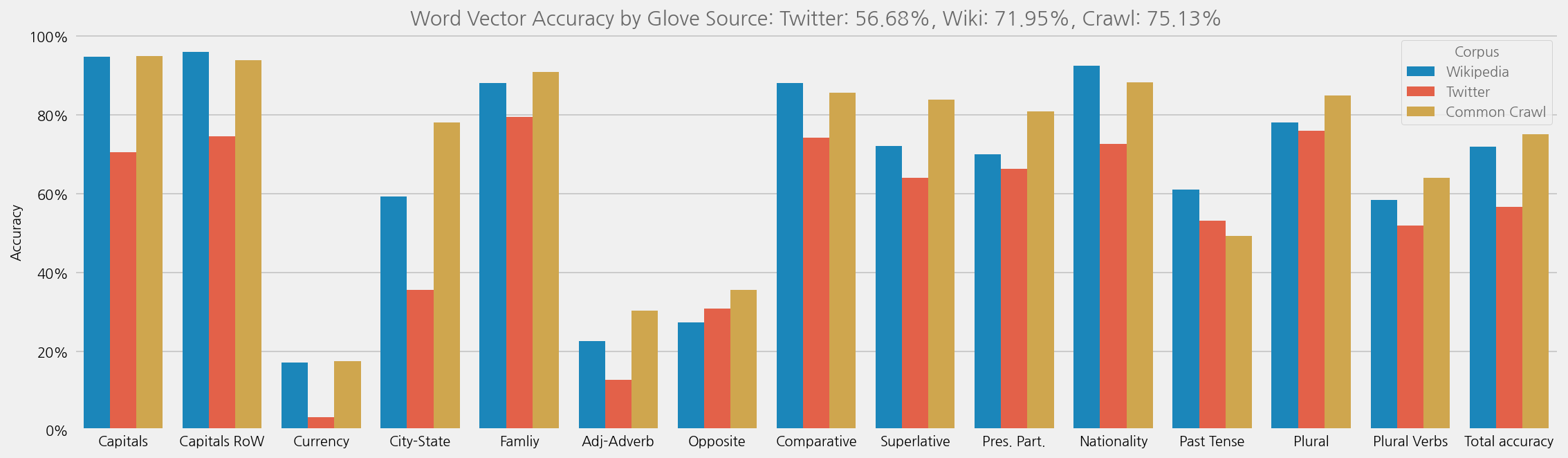
Visualize Embeddings#
results = wv_wiki.reduce_embeddings_2d(restrict_vocab=100_000)
vectors = results["vectors"]
word2idx = results["word2idx"]
INFO:ekorpkit.models.embeddings.wordvec:dimensions: (100000, 300)
INFO:ekorpkit.models.embeddings.wordvec:explained variance: [0.02604632 0.01293811]
best_analogies = wv_wiki.find_most_similar_analogies(
wiki_results["correct"], word2idx, vectors
)
best_analogies
wordpairs | word2idx | similarity | |
---|---|---|---|
category | |||
Adj-Adverb | (fortunate, fortunately, lucky, luckily) | (11156, 11584, 5065, 19955) | 1.998511 |
Capitals | (london, england, paris, france) | (516, 563, 1035, 387) | 1.780721 |
Capitals RoW | (vienna, austria, brussels, belgium) | (4094, 2640, 3879, 2975) | 1.996072 |
City-State | (chicago, illinois, omaha, nebraska) | (1147, 2884, 12159, 6087) | 1.971419 |
Comparative | (long, longer, heavy, heavier) | (173, 1078, 1106, 11613) | 1.932268 |
Currency | (usa, dollar, russia, ruble) | (2396, 678, 412, 17506) | 1.834880 |
Famliy | (sons, daughters, stepfather, stepmother) | (2912, 4321, 20624, 26903) | 1.999805 |
Nationality | (switzerland, swiss, israel, israeli) | (2311, 1849, 315, 406) | 1.999926 |
Opposite | (tasteful, distasteful, likely, unlikely) | (43255, 41259, 647, 2993) | 1.954353 |
Past Tense | (sitting, sat, hitting, hit) | (2995, 3223, 3141, 416) | 1.999999 |
Plural | (eye, eyes, donkey, donkeys) | (2090, 2251, 20328, 35193) | 1.999876 |
Plural Verbs | (talk, talks, estimate, estimates) | (1077, 370, 3470, 2886) | 1.739114 |
Pres. Part. | (write, writing, read, reading) | (2432, 1649, 1465, 2185) | 1.999959 |
Superlative | (weak, weakest, big, biggest) | (2690, 15655, 365, 882) | 0.663122 |
Total accuracy | (sitting, sat, hitting, hit) | (2995, 3223, 3141, 416) | 1.999999 |
Plot Analogy Examples#
wv_wiki.plot_similar_analogies(best_analogies, vectors, ncols=3, figsize=(15, 15))
INFO:ekorpkit.visualize.plot:No data to plot
INFO:ekorpkit.visualize.plot:No plots to plot
INFO:ekorpkit.visualize.plot:Saved figure to ./figs/plot_plot.png
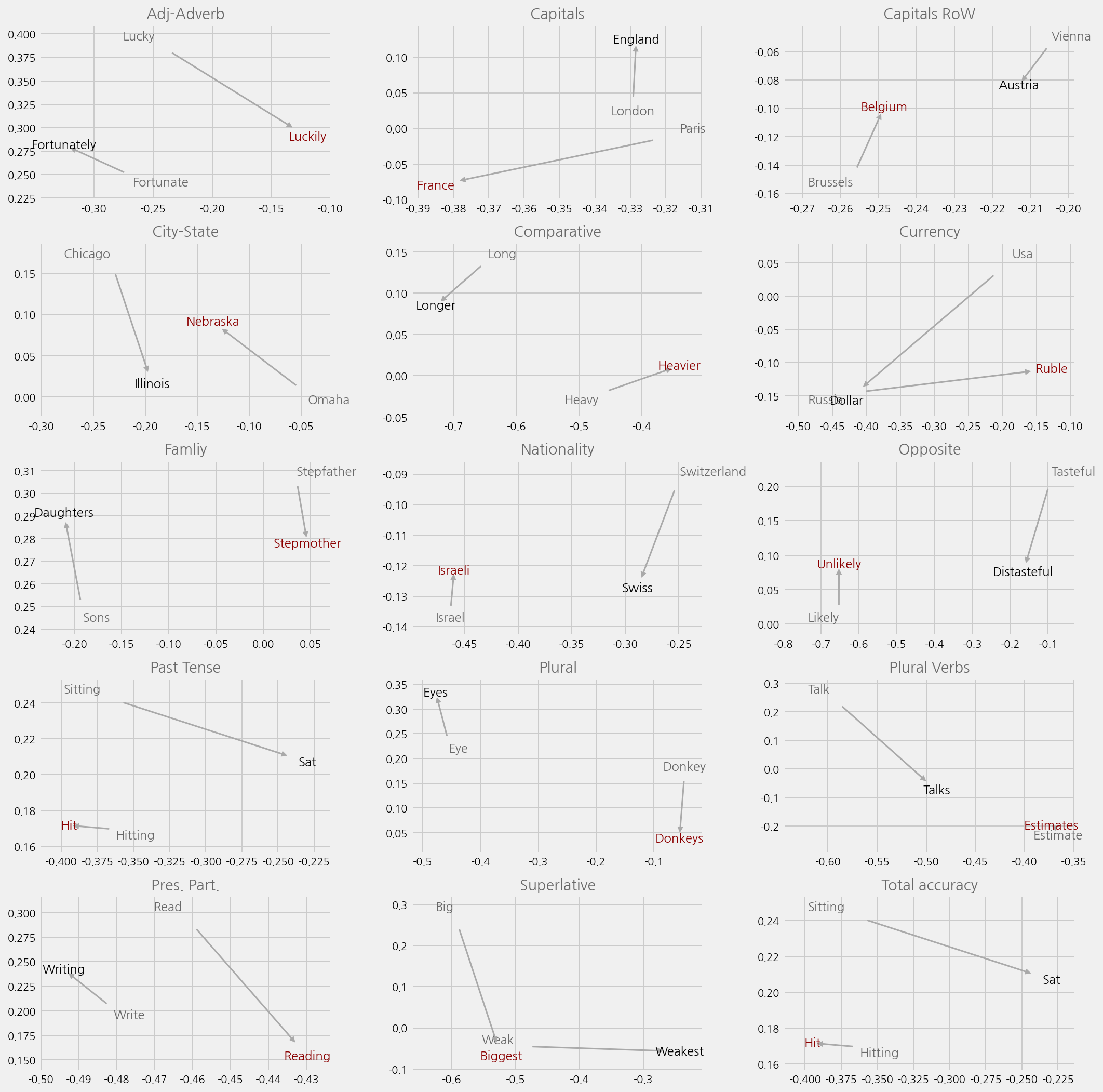